“We’re entering a trust crisis": Shifting left to scale agentic AI
What can be done to build up enterprises' confidence in AI and move from experimentation to implementation?
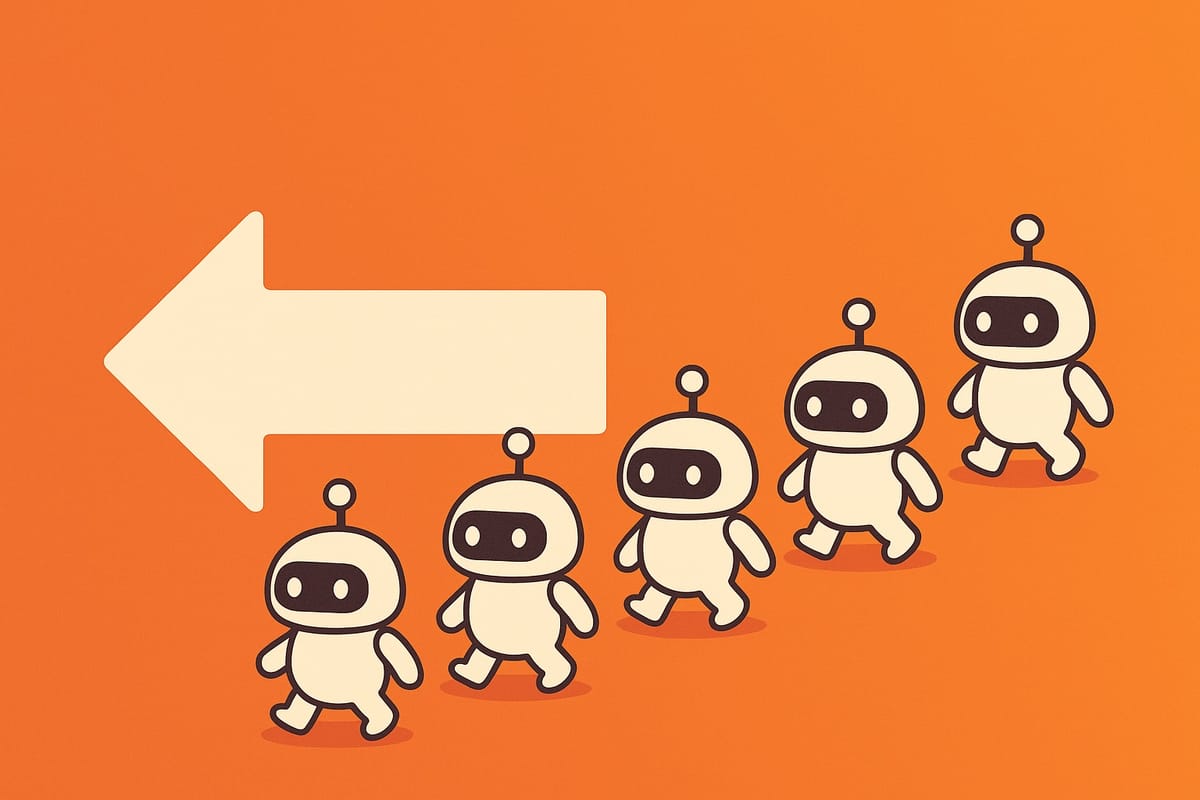
Developers are familiar with the concept of shifting left to catch bugs, drive up quality and integrate security right at the beginning of the development lifecycle.
By moving these critical tasks closer to the stage at which code is actually written, tech teams get faster feedback, can lower costs by catching issues early and will ultimately improve the final product by building in reliability and security from the start.
Could a similar process help to increase trust in agentic AI and help this game-changing technology scale in the enterprise?
To answer this question, let's start with the problem - which is abundantly clear. Neither businesses nor the public has full confidence in AI, meaning its benefits are being left on the table.
A recent study from IDC found that 80% of businesses have invested in agentic AI and are planning to integrate it into their workflows. However, just 12% feel ready to support autonomous decision-making at scale.
“We’re entering a trust crisis in AI,” said Nina Schick, founder of Tamang Ventures. “From deepfakes to manipulated content, public confidence is collapsing.
"If businesses want to build AI that scales, they must first build systems the public believes in. That requires authenticity, explainability, and a deep understanding of the geopolitical risks of unchecked automation."
Why don't businesses trust AI?
The Qlik AI Council - an assembly of artificial intelligence leaders convened by the data integration company Qlik - has been considering the issue of trust in an agentic context.
It warned: "AI that can’t be trusted won’t scale - and AI that can’t scale is just theatre."
Extrapolating a little from this statement leads us to the conclusion that businesses need to focus on building trust into their models right at the beginning of development - because when there is no faith in agentic AI, there is no chance of delivering its transformative impacts.
“AI that operates without transparency and redress is fundamentally unscalable,” said Dr. Rumman Chowdhury, CEO of Humane Intelligence. “You cannot embed autonomy into systems without embedding accountability.
"Businesses that fail to treat governance as core infrastructure will find themselves unable to scale - not because of technology limits, but because of trust failures."
Although the Qlik AI Council didn't explicitly call for AI trust work to be shifted left, its discussions reminded us of the classic DevOps mantra.
Build in trust at the beginning of the process and the outcome will be significantly better as a result.
The problem with agentic AI in the enterprise
The Qlik Council warned that agentic AI has barely made it out of the lab at most enterprises, which are justifiably concerned about bias, hallucinations and the ever-watching eyes of regulators.
This sluggishness is creating a new market reality in which competitive advantage is shifting not to companies with the most advanced models, but to those who can "operationalise AI with speed, integrity, and confidence".
“The market is short on execution,” said Mike Capone, CEO of Qlik. “Companies aren’t losing ground because they lack access to powerful models. They’re losing because they haven’t embedded trusted AI into the fabric of their operations. If your data isn't trusted, your AI isn't either. And if your AI can’t be trusted, it won’t be used.”
The Council called for trust to be designed into models (or shifted left, you might say) rather than being added later or rushed out hurriedly after a problem is identified.
It described execution as "the new differentiator", warning that agentic AI only works properly when its data, outputs and infrastructure are verifiable, explainable, and actionable.
The importance of trusted data
One of the foundational tasks organisations need to get right at the earliest stages of AI development is building datasets that are reliable, up-to-date and accurate.
Dr. Michael Bronstein, DeepMind Professor of AI at the University of Oxford, said: "Data is the lifeblood of AI systems, and not only do we need new data sources that are designed specifically with AI models in mind, but we need to make sure that we can trust the data that any AI platform is built on."
As businesses rush to turn experimentation into execution, new risks are emerging. Meanwhile, regulators are sharpening their pencils and preparing to introduce yet more rules to govern and control fast-moving AI technology.
“The regulatory landscape is moving fast and it’s not waiting for companies to catch up,” said Kelly Forbes, Executive Director of the AI Asia Pacific Institute. “Executives need to understand that compliance is no longer just a legal shield. It’s a competitive differentiator. Trust, auditability, and risk governance aren’t constraints - they’re what make enterprise-scale AI viable."
How can businesses build up confidence in AI, get the data foundations correct and avoid compliance nightmares? Shift those vital trust-building tasks left so that enterprises can have total faith in their model's accuracy, reliability and ability to make useful decisions autonomously. That's our opinion, anyway, and arguably the subtext of the Council's proclamations.
To hear more from the Qlik AI Council, join the Qlik Connect event this week in person or via livestream. Visit qlikconnect.com for more details.
Have you got a story or insights to share? Get in touch and let us know.